The Hybrid Renewable Energy Forecasting (HyREF) solution combines big data analytics and weather modeling technology to accurately forecast the availability of wind power and solar energy.
IBM's Hybrid Renewable Energy Forecaster
Rolf Gibbels | IBM Energy & Utilities
What does the Hybrid Renewable Energy Forecaster (HyREF) do and what makes it innovative?
The Hybrid Renewable Energy Forecasting (HyREF) solution combines big data analytics and weather modeling technology to accurately forecast the availability of wind power and solar energy.
The solution:
- Deploys high-resolution weather forecasting models to predict wind and solar energy generation.
- Applies advanced data assimilation, turbulence analysis and wake models, dynamically using real-time data from sensors to adjust forecasts in a 200m x 200m-scale region.
- Uses advanced physical and statistical combined power forecasting models for better generation forecasts.
- Applies advanced cloud imaging technology and sky facing cameras to track clouds in near-real-time, to adjust forecasts for solar power output and improve accuracy.
- Has sensors on the turbines to monitor wind size, strength, speed, temperature and direction.
What makes this solution innovative is its level of accuracy. When combined with analytics technology, the data assimilation-based solution can produce accurate extra short-term power forecasting (0-4h/per 15 min), short-term power forecasting (4-72h), and near-term power forecasting (1 month) within a wind farm. Additionally, HyREF can also predict the power output of a single wind turbine, which in turn can reduce forecast errors to less than 10 percent.
From a solar perspective, HyREF uses cloud image analytics and advanced numerical prediction models to forecast cloud movements and their impact on solar panel radiation and also can precisely calculate other weather impacts on solar generation.
Does the solution work in all parts of the world or only in certain climates?
The HyREF solution can be applied anywhere in the world and is independent from certain climates. HyREF can be adapted based on data availability (historical and actual), which can vary depending on a country’s or region’s sensor infrastructure (existing or new), publicly available data or wheter, in the case of wind, the project is onshore or offshore.
Does HyREF only work on existing wind turbines or can it be used in the planning stages of a wind farm?
HyREF is used both with existing wind installations and for planning purposes. For example, during the planning stage, it is critical to determine the best location for each wind turbine, taking into consideration not just the weather but also the terrain and how each turbine impacts the other next to it. If turbines are placed too close to each other, it can create drag or a ‘wind shadow’. HyREF assists with careful planning, so turbines don’t slow each other down and so that the impact of many turbines on a wind farm is well understood before any construction and power capacity estimates are provided for financing.
Discuss how the granularity of forecasts enabled by HyREF – down to the individual turbine – will benefit a wind farm operator?
Understanding the behavior and characteristics of each turbine is beneficial to the operator for many reasons. For example, to best manage each turbine maintenance schedule, it is important to know the unique stresses placed on the turbine through various wind speeds, too much wind (need to shut down), or impact from other turbines through drag. This will allow the operator to most effectively run each turbine against demand requirements and availability.
How can a utility benefit from HyREF, especially one that’s coal-based?
HyREF’s high degree of accuracy (90-94 percent) allows a utility with the proper smart grid and transmission infrastructure to optimize the use of wind power and avoid unnecessary curtailment. In turn, this allows the use of conventional sources such as a coal to be minimized and also allows the utility to avoid some of the redundancy costs associated with running back-up coal generators, since there’s a much greater understanding of the amount of wind power that can be expected in a given timeframe.
Describe your ongoing implementation with State Grid Jibei Electricity Power Company Limited. What are some key learnings and outcomes you’ve seen initially?
State Grid Jibei Electricity Power Company Limited (SG-JBEPC), a subsidiary company of the State Grid Corporation of China, is using HyREF to integrate renewable energy into the grid. This initiative led by SG-JBEPC is phase one of the Zhangbei 670MW demonstration project, the world's largest renewable energy initiative that combines wind and solar power, energy storage and transmission. This project contributes to China's 5-year plan to reduce its reliance on fossil fuels.
By using the HyREF wind power forecasting capabilities, a long-term accuracy level of 90 percent was achieved and 10 percent more energy was delivered to the grid. This amount of additional energy can power more than 14,000 homes. We are continuing to collaborate with SG-JBEPC to gain additional insights.
How do you envision HyREF impacting the renewable energy industry?
The renewable energy industry is still a young industry that over the last decade has experienced strong growth. Wind energy represents the lion’s share of all new capacity and with that positive growth came new challenges, such as renewable integration with the grid and other conventional sources, increasing operations and maintenance costs, and curtailment.
By using HyREF, these challenges can be better understood, then analyzed and optimized. This starts with planning the best location for the generation site and continues with advanced and accurate weather and power forecasting. In general, topics such as analytics, big data and cloud will play an increasing role in positively impacting the sustained growth of renewables.
HyREF will also impact clean energy policies and climate plans. For example, countries like China require forecasting at a particular accuracy level to meet output levels and avoid fines and regulatory consequences. Accurate planning and forecasting of performance at a future generation site has a direct impact on the financing model and is a key factor as to whether a project will be approved or not.
About Rolf Gibbels, Global Power Generation Solutions Leader,
IBM Energy and Utilities Industry
Rolf Gibbels is an executive member of IBM’s global Energy & Utilities organization and leads the global industry solutions and business development activities for power generation, including renewables, fossil and nuclear energy sources, across the services, software and infrastructure groups.
Rolf has more than 15 years of international experience selling and marketing industry software solutions, product lifecycle management (PLM) software, and computer aided design (CAD) software to the energy, process, architecture and construction industries.
Prior to IBM, Rolf was Director of Global Market Development and Energy Industry Solutions at Dassault Systemes and Vice President-Sales at Bricsnet. He holds a master’s degree in Civil Engineering from the University of Applied Sciences in Munich, Germany.
The content & opinions in this article are the author’s and do not necessarily represent the views of AltEnergyMag
Comments (0)
This post does not have any comments. Be the first to leave a comment below.
Featured Product
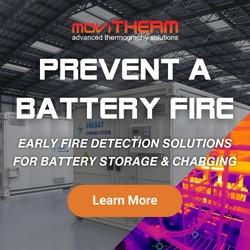