While other solar forecasting systems take more narrow location and timeframe views, whats different about the IBM approach is that we incorporate a great number of weather and solar energy prediction models. We then blend those using historical data as a function of weather situation, forecast horizon and location to create what we call a supermodel.
Hendrik Hamann | IBM
IBM unveils results that show its scientists can generate advanced forecasts of solar energy that are as much as 30% more accurate than other state-of the art approaches.
What is the purpose of IBM’s collaboration with the U.S. Department of Energy? Who will it impact?
IBM’s goal is to help produce a more sustainable energy future by integrating solar power into the energy pipeline. Our collaboration with the DOE’s SunShot Initiative now makes this possible as this national collaborative effort seeks to make solar energy fully cost-competitive with traditional energy sources before the end of 2020. We have demonstrated that solar and wind forecasts can be as much as 30 percent more accurate than traditional approaches. At this time, the two main customers: utility companies and independent system operators (ISOs).
Who are you working with to make this a reality?
Through the DOE Sunshot Initiative, we are working with a number of collaborators in academia, government and industry to gain various perspectives. For example, NREL and ISO-New England are two main partners who are helping evaluate the important metrics to determine accuracy of solar forecasting. Also, for a limited time, IBM will provide foundational solar forecasts covering all 48 contiguous states at five kilometer spatial resolution. This will primarily be for government agencies, utilities and grid operators to evaluate how solar forecasting can impact the supply and demand, in addition to operations.
How does it all work?
The forecast technology uses a combination of machine learning, big data analytics and mathematical modeling of complex weather systems. The system continuously monitors weather conditions, including satellite observations, and analyzes that data to forecast how much solar energy will be available at different locations and times.
What sets the IBM approach apart from other forecast technologies?
Think of this as big data meets science. While other solar forecasting systems take more narrow location and timeframe views, what’s different about the IBM approach is that we incorporate a great number of weather and solar energy prediction models. We then blend those using historical data as a function of weather situation, forecast horizon and location to create what we call a supermodel. These advances are valuable for the future of alternative energy. We have shown we can generate accurate forecasts of solar energy from minutes ahead to even several days.
How will the data be accessed by those seeking to use it for solar system installations?
The data will be accessed via the cloud as a data service. Organizations across the nation can log into the website to get foundational solar forecasts.
What do you believe is the future of alternative energy?
At IBM, we’re convinced that with better forecasts and supporting efforts, it’s possible that solar energy’s contribution to the United States’ energy will reach up to 50 percent. Until now, most experts agree that because of intermittency, solar energy won’t supply more than 20 to 30 percent of the United States’ energy. As we continue to refine our system in collaboration with the DOE, we hope to double the accuracy of the system in the next year. That could have a huge impact on the energy industry – and on local businesses, the economy and the natural environment. Part of our goal is to help a wide range of industries and professions better understand how the world works so we can all make better decisions.
Are there other areas of research and development that IBM is working on with respect to the future of alternative energy?
Yes, three years ago, IBM Research formed the Smarter Energy Research Institute - a consortium of energy and utilities companies looking to transform their business through the use of advanced computation, predictive analytics and system optimization. Part of these efforts include helping the organizations meet renewable integration and distributed energy resource regulatory targets while ensuring system stability: http://www-03.ibm.com/press/us/en/pressrelease/44202.wss
About Dr. Hendrik Hamann
Dr. Hendrik Hamann is a Research Manager at the IBM T.J. Watson Research Center, Yorktown Heights, NY. He received his PhD from the University of Goettingen in Germany. Since 2001 he is leading the Physical Analytics program in IBM Research, as a Research Manager. His expertise includes sensor networks, sensor-based physical modeling, oil and gas, renewable energy, precision agriculture, energy management and system physics. He has authored and co-authored more than 70 scientific papers and holds over 85 patents. Dr. Hamann has served on governmental committees such as the National Academy of Sciences.
The content & opinions in this article are the author’s and do not necessarily represent the views of AltEnergyMag
Comments (0)
This post does not have any comments. Be the first to leave a comment below.
Featured Product
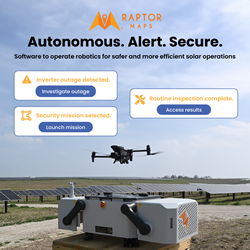