Batteries may degrade gracefully up to a point, then experience “rapid fade” and lose capacity quickly. A system that has been designed to meet a given level of demand will, at some point, be unable to do so.
Understanding Battery Degradation
Paul Hofmann | SpaceTime Insight
Energy storage is a key component in making renewable energy competitive with fossil fuel generation. What has to happen for batteries to be part of the solution?
First and foremost, batteries need to be cost-competitive with the alternatives. Storage is an integral part of intermittent renewable power generation, be it wind or solar or another source that depends on environmental conditions. Today, it is still more cost-effective to fire up a gas peaker plant than to use battery storage when renewable energy sources can’t meet demand. Kinetic energy systems (e.g. pumping water or driving weight uphill during daytime, solar producing hours and using it to drive generation overnight) are also available. Long term, however, batteries should present a clean, efficient way to instantly and sustainably balance supply and demand in renewable energy systems. Driving the costs of battery storage down requires better battery management systems, including better analytics to predict capacity degradation and help optimize charging to extend the life of these assets. Machine learning models will be at the heart of these systems. They can see beyond the engineered characteristics of the battery units to each unit’s actual operation, and combine that with usage profiling to generate far more accurate predictions of capacity over time. Machine learning models will also optimize the operation of the battery storage systems, improving financial outcomes for all players in the grid.
Battery storage is becoming the future storage of choice since we have reached the crossover point where renewable power generation has become cheaper than coal generation. For example, ViZn Energy Systems (a safe energy storage company) claims it can pair a solar power plant with an energy storage system for 4 cents per kilowatt-hour (kWh). Pairing its 30 mega watt (MW), 4-hour duration zinc-iron flow battery with a 100 MW solar plant can generate a 7 percent internal rate of return – all under a 4 cents per kWh power purchase agreement. ViZn says energy storage can now be added to a wind farm or solar plant at a lower price than new coal-fired generation based on 6 cents per kWh cost.
How does battery capacity degradation impact battery operations? What are some of the key impacts on battery degradation?
Batteries do one thing: store and release energy. Any battery unit has to be able to store and release a predictable amount of energy to be part of a functioning energy storage system. All batteries lose capacity over time. Unfortunately, this degradation is not linear. Batteries may degrade gracefully up to a point, then experience “rapid fade” and lose capacity quickly. A system that has been designed to meet a given level of demand will, at some point, be unable to do so. Battery manufacturers provide a warranty that a unit will provide a certain capacity for certain period of time, but without the right models to predict degradation over time on a unit by unit basis, they must accrue excess funds for the cost of replacement. End users must account for the uncertainty by planning excess capacity or otherwise allow for experiencing capacity reduction while units are replaced. All of this adds to the cost of operation. Degradation is not a straightforward engineering problem. Battery chemistry is affected by temperature and charge and discharge cycle patterns. These, in turn, are affected by the unit’s location and the behavioral profile of the customer using the battery. The best way to really understand what is happening with a given battery unit is through a continuous machine learning model that is able to predict the degradation as far in the future as needed with a known confidence interval in order for the prediction to be meaningful.
Are some battery technologies better than others as far as degradation is concerned?
Battery chemistries behave differently. While we’ve focused on lithium batteries and can discuss that technology’s characteristics, the methods we use to understand how they degrade would be the same across battery types. Different technologies may be appropriate for different use cases, but optimizing batteries is important for all use cases.
Don’t battery manufacturers understand how their batteries degrade? What does machine learning bring to the table?
Of course, the manufacturers do have a great deal of in-depth knowledge about how their batteries work. Like any manufactured asset they’ve done their best to engineer certain characteristics into the product. With their knowledge of the asset’s design, they can make general predictions about how the battery should degrade over time.
Machine learning improves upon engineering know-how into two critical respects. First, using unsupervised machine learning, we can let the analytics discover the hidden variables that lead to failure. The engineers only know about a few identified variables, but machine learning can discover what combination of physical and chemical states leads to a higher probability of failure. These may represent hidden (and sometimes seemingly insignificant) manufacturing defects, or other affects that were not intentionally engineered into the product but nevertheless have an impact on degradation. By discovering these hidden states and watching the battery transition back and forth between them we can create a better model of the true probability of the failure time for a specific unit, not just a class of assets like a model or a manufacturer.
Second, as noted above, how the battery is used affects the degradation. The manufacturer can’t know in advance how a battery will be charged and discharged on a per-unit basis. They can only create models based on averages. However, using machine learning we can learn the usage profile of a particular customer over time, and then predict how that customer will use that particular battery in the future. This adds accuracy to our models. Additionally, we are able to work with censored data using unsupervised learning. For context, censoring is a condition in which the value of a measurement or observation is only partially known in statistics, engineering, economics and medical research. Only a certain class of algorithms can properly handle censored data. Most machine learning algorithms, including deep learning, would have to leave valuable information out from censored data since they cannot learn directly from it. Combining these unsupervised algorithms with the physical model gives us a very rich probability distribution that allows us to predict the capacity degradation with great confidence into the future.
Why is it critical to better predict capacity degradation?
If you are deploying utility-scale energy storage, it is critical to understand the capacity you are likely to lose over time; your ability to meet demand at any time is dependent on a complete understanding of your available supply. Regulated utilities in particular need to plan and gain approval for capital expenditures.
Another important reason is to help create better financial models that ultimately reduce the cost of battery systems. Take, for example, residential battery storage units deployed in conjunction with rooftop solar systems. In order to sell such systems, the manufacturer might warrant that the batteries will continue to hold at least 50 percent of their capacity for ten years. If they haven’t been selling those systems for at least that long, they have no real history to help them model their warranty liability. Manufacturers have some laboratory data they can extrapolate from, but battery charge and discharge cycles in the wild are very different from the ones in the lab. They will have to accrue surplus funds to mitigate the risk of replacing wrongly predicted units during the warranty period.
A better model would tell manufacturers how many units are actually likely to fail each period. Using the failure time prediction, they can optimize their financial risk calculations based on reinforcement learning models that can take into account constantly changing variables like the market prices of manufacturing inputs and inventory levels. Manufacturers will be able to accrue a far more realistic and smaller amount of money for warranty replacement. This more realistic picture saves them millions of dollars in the cost of capital as it frees up funds for use elsewhere. Further, the cost of operation for battery storage can be significantly reduced if you add to this the savings available all along the operational chain by optimizing maintenance schedules, improving charge/discharge cycle practices, monitoring customer usage profiles and more.
What are some other problems in the alternative energy marketplace that this type of machine learning addresses?
Besides better predicting battery degradation for the smart grid, there is another prediction problem for photovoltaic (PV) power plants, solar power inverter degradation. Generally, inverters will fail before the panels do. So, inverters have a significant impact on the operating cost of the system. In another financial modeling use case, many solar systems are financed. Built into the cost of financing is a certain probability that the system will fail. In the solar market, many installed systems use low-cost panels and inverters with unknown reliability. Financing can be offered at lower rates to bring the total cost of ownership in a solar system down by using machine learning analytics software to monitor and predict failure times on a unit-by-unit basis, as well as using reinforcement learning to optimize the financial models for the whole PV field with its complex interactions. As with batteries, making alternative energy cost-effective is critical to adoption.
Unsupervised machine learning systems, like hierarchical Bayesian networks, have an advantage over condition-based maintenance systems or even supervised machine learning systems in their ability to learn the “unknown unknowns.” This means that the unsupervised machine learning system is not dependent on a detailed engineering model of the asset it is learning about, and it can model causality since it learns about hidden variables. So, it can use a battery degradation model on a variety of battery manufacturers or models. Similarly, the same set of machine learning libraries can be used to model wind turbine gear boxes alongside batteries and solar inverters. These models must be built for the type of asset being analyzed, but the principles are the same and extensible across a wide variety of asset types.
Reinforcement learning is used to find the optimal policy given an objective function in a financial system, like a given risk for the warranties. Making decisions according to the optimal policy is lowering maintenance costs, warranty accruals or financing interest rates.
So, failure time prediction and operational and financial optimization models will help improve operating costs for wind turbine gearboxes, hydropower turbines, batteries and more.
The NREL recently reported that up to 5 million businesses could benefit from behind-the-meter battery energy storage. How might understanding capacity degradation help these businesses better take advantage of storage technologies?
The NREL report points out that regulated utilities often have high-peak demand rates that are offset by lowered volumetric rates. Businesses that are able to incorporate energy storage to avoid peak demand charges will benefit from the lower volumetric rates on the majority of their electrical usage. Along with other benefits, this rate play can make energy storage a smart investment. Of course this depends on making the numbers work. All of the positive impacts machine learning can have on the bottom line cost of operating battery storage come to bear on that simple equation: does this make financial sense? With the right analytics working behind the scenes, increasingly the answer will be, “Yes.”
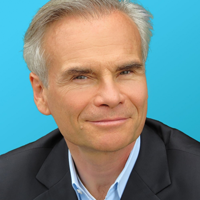
Paul Hofmann, PhD. draws on over twenty years of experience in enterprise software, analytics and machine learning to lead SpaceTime Insight's analytics and data science strategy. He has held executive roles at BASF and SAP, where he was VP R&D, and conducted academic research at MIT, Technical University in Munich and Northwestern University. Most recently, Paul served as CTO for Saffron Technology, now part of Intel.
The content & opinions in this article are the author’s and do not necessarily represent the views of AltEnergyMag
Comments (1)
Featured Product
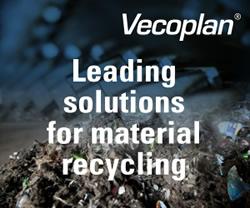